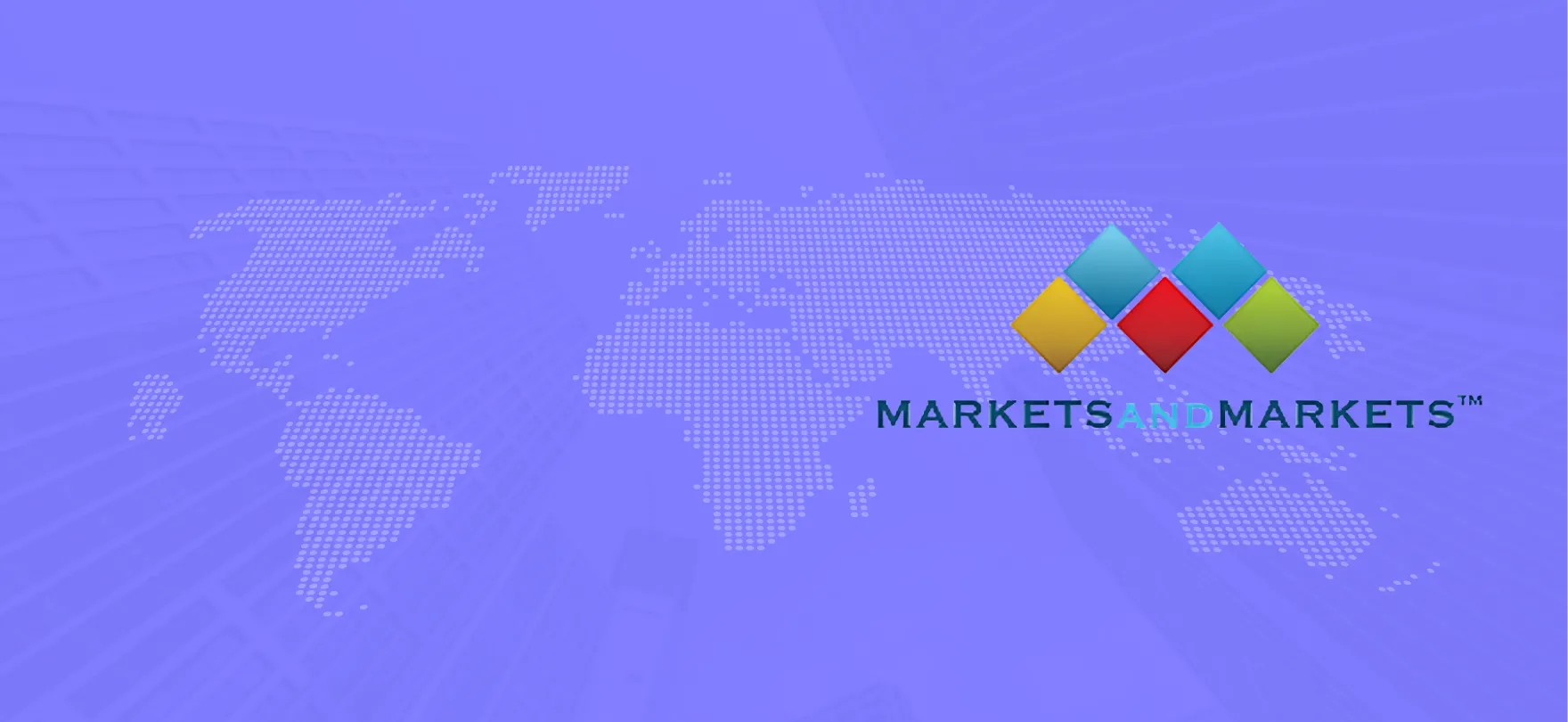
How Face Biometric Screening Would Be the Game-Changer of the AML Industry?
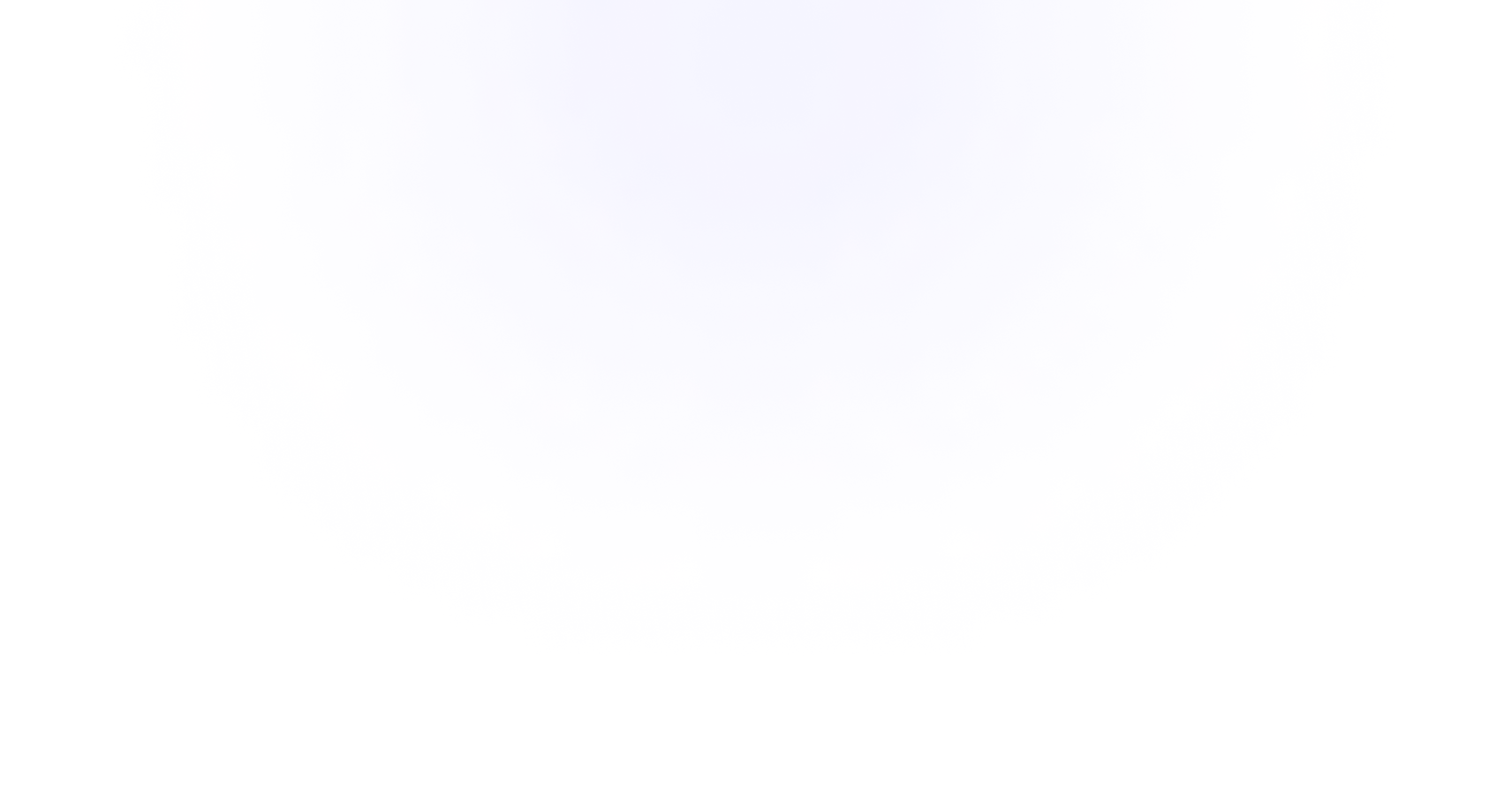
The domain of AML is unreservedly very complex where AML tools together with extensive data are dealt with. In such a complex field, particularly the financial sector faces a huge challenge. One big and persistent challenge is the occurrence of false positives (erroneously showing a legitimate person as someone who might fall on a watchlist, sanctions lists, and even as a PEP profile). Such errors pose two kinds of problems for organizations and financial institutions. Firstly, the resources for verifying the case are wasted because of the misassessment of the risk. Secondly, a potentially valuable customer to the banks might be lost because a fault in the AML tools might portray him/her as a high-risk individual when he/she is not. Consequently, financial institutions with low-risk appetites may decide to refuse onboarding those customers, leading to a loss in business. Apart from false positives, updating the database is another challenge. This is because the results ought to be produced in real-time and the organizations may onboard a fugitive or a fraudster because the database was not updated.
Face Biometrics – The Solution
To counter the major challenges mentioned, the tool of face biometric screening greatly solves the problem. This tool has revolutionized the conventional AML practices. When the image of the person is uploaded, it searches and matches the face in the vast database of watchlists, sanctions lists, and PEPs. If the person exists in any of the databases, an exact match is shown which greatly reduces the rates of false positives. This is because the results or matches are accurate and very precise. Additionally, since the face search happens efficiently, time is also saved. Surprisingly, this innovative approach is not yet widely adopted or explored in the AML domain.
Adoption of the approach can lead to face biometric screening ascending to become the cornerstone of AML with its potential to offer a more efficient, accurate, and technologically advanced solution to the persistent challenges in this field.
Understanding Face Biometric Screening
The way face biometric technology works is by utilizing highly advanced facial recognition algorithms to identify even the most unique facial features. The name to be checked on the watchlists or sanctioned lists is written and the image (which acts as a unique identifier) is uploaded. The face in the picture is then matched with the huge dataset of faces in the watchlists, sanction lists, and PEPs.
The facial match identifies the exact person in the lists in which he/she may lie and this greatly reduces the conundrum of false positives or negatives which is the pain point that leads the clients to switch the AML providers they have been using.
The market of face biometrics and face matching is growing at an exceptional rate. This is supported by a report by MarketsandMarkets, that the facial recognition market is projected to reach $8.5 billion by 2025.
The Distinction Between Traditional AML Methods and Face Biometric Screening.
Traditionally, AML algorithms have long relied on unique identifiers like name, date of birth (DOB), and nationality to ensure fast and accurate identification and match. However, these methods have notable limitations.
- Firstly, such credentials require verification and re-verification from multiple documents and platforms which can be very time-consuming. Such practices cause inefficiencies.
- Since the DOB and nationalities are unique identifiers, any wrong input, or inconsistencies or errors even in the documents can lead to wrong identification of the person. Additionally, people of the same names can even have the same nationalities and this further produces confusion since in such cases nationalities can not act as unique identifiers. Hence, the scope of screening needs to be expanded in such situations.
- False positives can also result if there is some spelling mistake or variation in names, which can occur due to transliteration differences.
- Multilingual differences also pose a challenge since the names of other unique identifiers may appear differently in different languages. All of these challenges highlight that the conventional/traditional AML strategies have limitations, ultimately leading to wrong identification and misassignment of risks.
As opposed to the traditional ways of performing AML checks, face biometric screening offers a more direct and reliable form of identification.
This is because in this case, the unique identifiers are the facial features of the individual under checks. If the algorithms are strong and accurate, this technology can bypass many of the issues associated with traditional data fields. The rates of false negatives and positives are greatly reduced because face biometric screening can still match accurately regardless of the variations in how the data is recorded in the documents, differences in language, name spelling, or the possession of multiple nationalities. Since facial features are less prone to changes as compared to textual data, face biometric screening becomes the game changer in the AML industry.
Face Biometric Screening Example in the Law Enforcement
In the UK there are 13 biggest retail shops that in association with the police authorities are now part of the Retail Crime Action Plan. As per this plan, the retailers must submit images of CCTV in which people involved in shoplifting are taken. The images that are submitted are matched through the Police National Database. In this facial scanning is used to match the image of the individual collected from CCTV with the images in the database compiled by the police, according to a report by BBC. Because of the use of this technology, as per the report of BBC, 350,000 shoplifting cases were reported and addressed in England and Wales.
Facial biometric screening has been used by Interpol with the development of its face recognition system known as the INTERPOL Face Recognition System (IFRS). Interpol’s database is unique since it contains facial images from 179 countries. These individuals exist in the watchlists. The system uses computerized facial recognition technology to identify or verify individuals of interest with the aid of analyzing facial features and contours. This technology was launched in 2016 and since then the IFRS has identified almost 1,500 terrorists, criminals, fugitives, persons of interest, or missing persons.
The Role of Artificial Intelligence and Machine Learning in Enhancing Face Biometric Systems in the Future
It is forecasted that the integration of AI and ML in face recognition technology will make the biometric tool more efficient and accurate, such that they can read or analyze the face under various circumstances (blurry picture, dark light, side of the face, etc). Since searches through faces have proven to be the best unique identifier with reduced rates of errors, notable advancements are seen in biometric screening encompassing multiple modalities. Interestingly, there is a window opening of another technology called liveness detection to counter spoofing attempts, ensuring that the possibility of entering the organization and its systems through a fraudulent or shady way is greatly minimized. The integration of blockchain in biometric databases, as explored by Biometrics and Data Pattern Analytics Lab, could enhance security and transparency, fostering trust in the authentication process. These various outbreaks in improving face-matching technologies will play a crucial role in making strides toward stronger and adaptive AML practices.
Best Practices
There is no doubt that face biometric screening has revolutionized conventional AML strategies to a great degree so much so that the most challenging issue of the high rate of false positives has been overcome. However, organizations must ensure that the right balance is striking between AML compliance and privacy and ethical concerns.
Transparency with Customers:
While the customers are being onboarded, it is imperative that they are explicitly being informed that their images will used for performing AML and KYC procedures. Doing this would ensure transparency which is a part of ethical business practices.
Compliance with Data Protection Laws:
Regulations such as the General Data Protection Regulation (GDPR) in the EU, the California Consumer Privacy Act (CCPA) in the US, and other local data protection laws must be followed because abiding by these laws ensures how personal data (which majorly includes biometric data) can be collected, processed, and stored.
Using Updated Data:
How accurate biometric screening is also relies on how frequently the data is being updated. Missing out on any high-risk person would mean that either the algorithm of the tool was faulty or the database was not updated timely.
Image Quality and Format:
The quality of the image used determines the result of screening. It must be ensured that the image being used is well-lit and very clear. Moreover, the size of the image must be correct to the required measurements. A poor-quality image can cause misidentification and errors in the results.
Example
An MLRO in a world’s financial hub like London may face difficulty in finding the true match due to a lack of familiarity with South American names. However, a face match through biometric data verification may make it easy to find the true positive.
Key Insights
As the problem of false positives and negatives becomes a persistent conundrum in the AML industry, biometric screening greatly decreases the occurrence of such errors with its ability to offer exact matches. The picture acts as a unique identifier of the individual and creates ease for the MLROs. This technology is fairly different from the traditional AML strategies. However, AML confronts challenges such as identity fraud, complex laundering schemes, and the need to comply with continuously evolving global compliance demands. The challenges are further aggravated by the increasing costs incurred on ensuring compliance as compliance costs are estimated between $700 million and $1.5 billion over the next decade (Thomson Reuters). These challenges pave the way for the integration of better technology like AI and ML into AML tools, especially biometric face matching. Many financial institutions despite the ethical and privacy concerns have been able to adhere strongly to the AML laws with the aid of biometric technology. For instance, the Royal Bank of Scotland’s adherence to the Fifth Anti-Money Laundering Directive with the huge investment into face matching signifies the efforts that can be made by other, rather new financial institutions. Additionally, Microsoft’s ethical stance on facial recognition technology highlights the need for responsible use of biometric data. From future perspectives, joint efforts to revolutionize the AML industry between industry leaders and policymakers are crucial for shaping adaptive AML practices. This can be achieved through integrating technologies like face biometrics with artificial intelligence, machine learning, and even liveness detection. For a sensitive industry, it becomes imperative to take proactive measures to protect not just individuals but organizations as well.
Related Articles
We are here to consult you
Switch to AML Watcher today and reduce your current AML cost by 50% - no questions asked.
- Find right product and pricing for your business
- Get your current solution provider audit & minimise your changeover risk
- Gain expert insights with quick response time to your queries