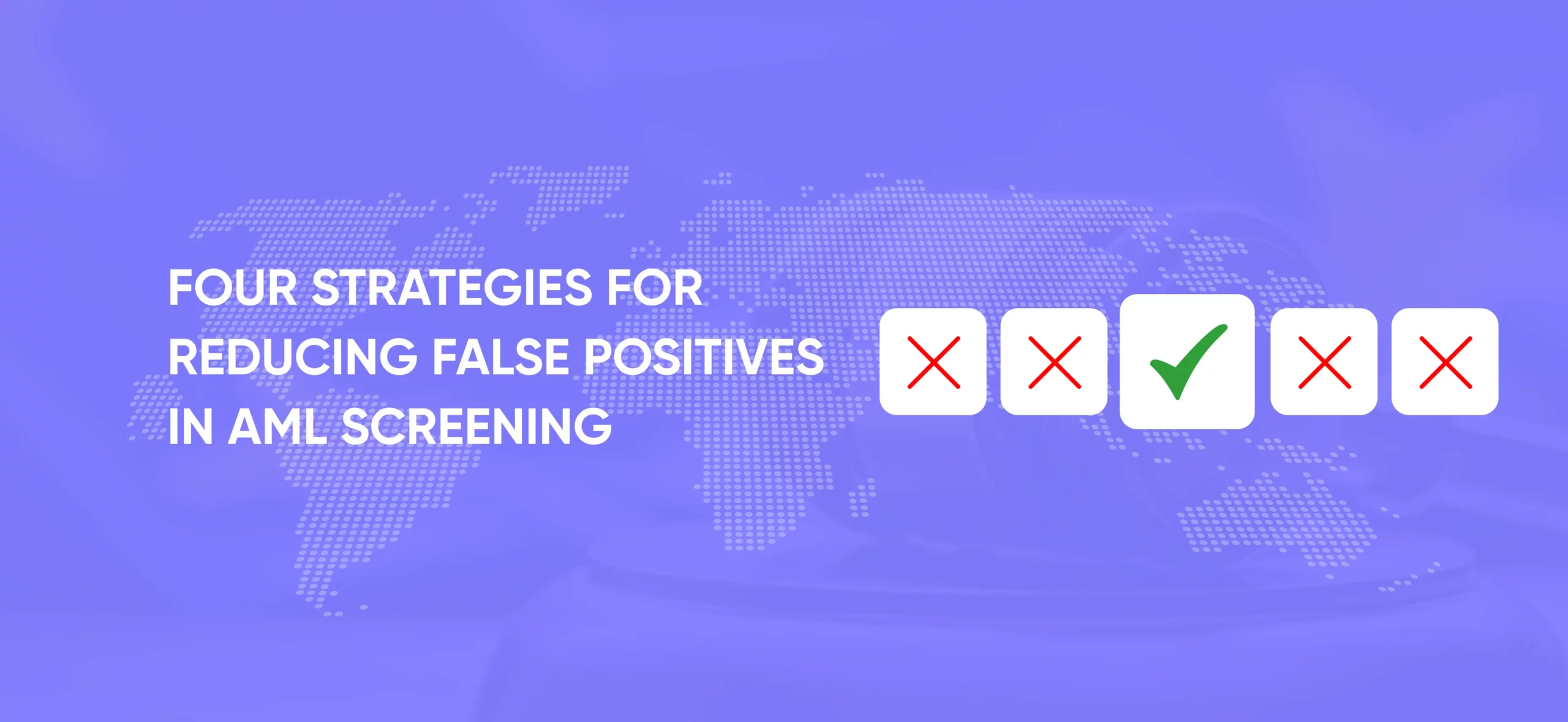
Top 4 Strategies To Reduce AML False Positives
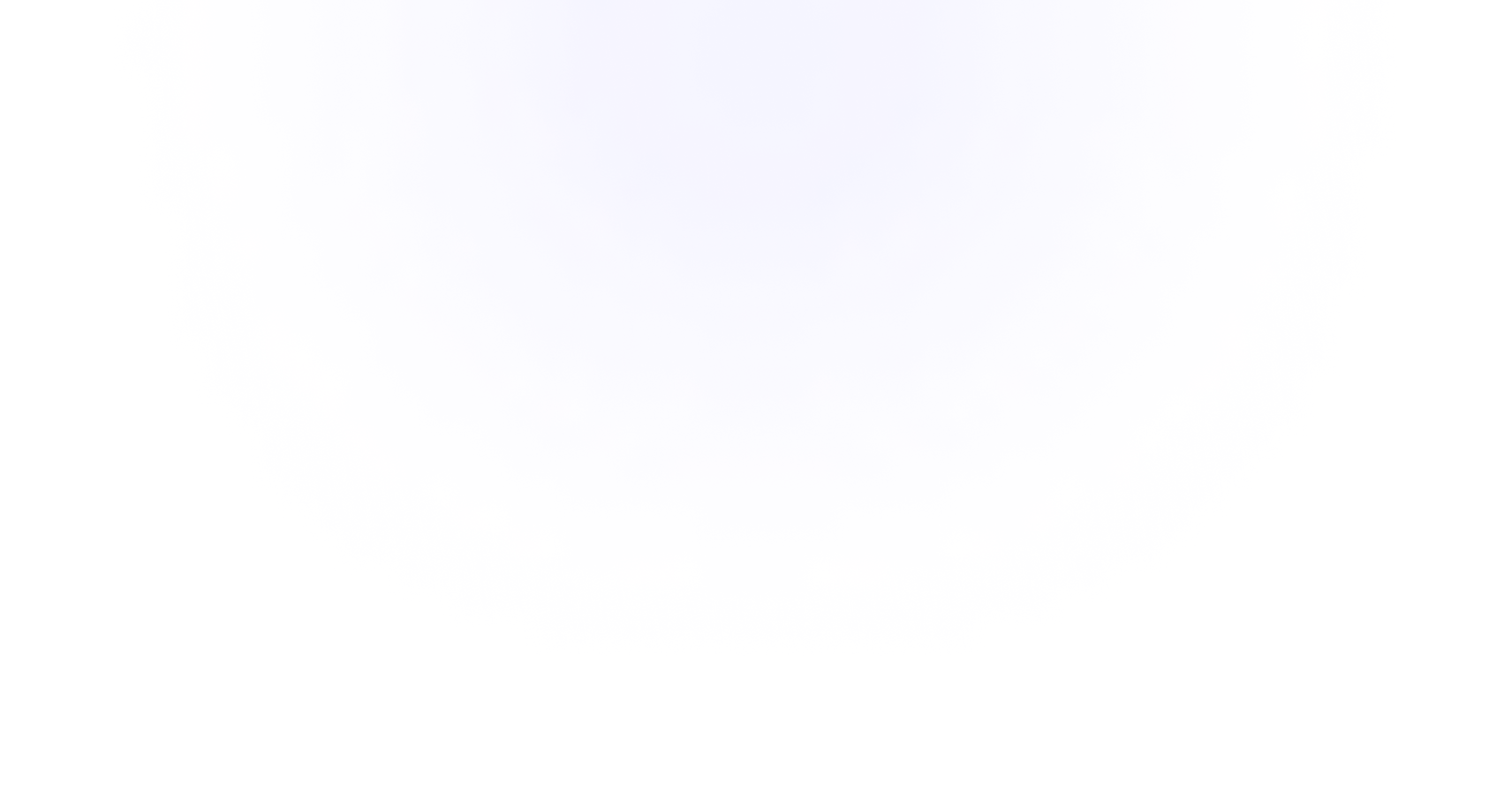
Envision a situation where your Anti-Money Laundering system refers to a security personnel of a high-profile event who is even more alert than a Hawk’s eye.
Every time someone gets closer, the guard’s instinct is to sound the alarm.
This is usually a very cautious approach that results in a lot of alerts actually turning out to be false alarms thereby causing a lot of confusion at the event.
False positives for AML screening work in the same way—the legitimate undertakings get caught in the crossfire, and the costs go up along with customers’ dissatisfaction.
Let’s comprehend the idea of false positives first and then get a detailed command on AML false positive reduction tips.
What Are AML False Positives In AML List Screening?
A false positive in AML compliance means spotting a customer’s name apparently matching a similar name from any global watchlist, sanctioned lists, or PEP data, that could turn out inaccurate after detailed analysis.
For instance, a system blocked transactions with “Global Holdings” based on a name that is similar to an entity on the sanctions list, say “Global Holdings Ltd.,” which turns out to not be the actual target.
This false positive check actually implies that one ties resources and time to investigate a legal transaction that is not all that risky.
Have you ever thought you are a high-net-worth individual and your daily transactions could be considered suspicious and flagged for money laundering?
Last year, Chase’s AML system made headlines by doing exactly that, flagging the genuine transactions of some of its most well-known customers as suspicious.
How did this false-positive challenge come about?
This false-positive disaster was realized because the system’s algorithm was oversensitive to responding to normal behaviors as risky.
This case shows that while it is extremely important to catch financial fraud, there is always great danger for percentage precision.
At present, it has been estimated that about 95 percent of such system-generated alerts are actually false positives.
Is biometric screening a right fit to reduce false positives? You may find out here!
Have you got a basic understanding of AML false positives? Now it’s time to move forward and uncover the secrets behind the higher number of false positive rates.
Why do financial institutions detect and report the right customers under the box of suspicious clients?
How Do AML False Positives Arise?
The AML false positive is totally different from a true positive described as a sanctioned entity that matches the searched name and cannot transact—and a true negative refers to a non-sanctioned entity or person that successfully passes through the screening.
By far the alarming AML screening failure is a false negative, which is approving of any sanctioned or blacklisted profile as a legal profile.
Thus, any “financial institution or obligated entity must distinguish between real positives and negatives as well as prevent maximum false positives or false negatives” to stay compliant.
The major reasons for the rising false positive ratios are:
Name Similarity: The Case of the Mistaken Identity
Think of a detective who is searching for criminals and ends up arresting anyone who shares the same name.
That is the core of the problems with name similarity in AML list screening!
Problems that come from name similarity happen where legal transactions or legal clients are blocked because they have names similar to those of persons or organizations known to be involved in some form of illicit activity or are on a sanction list.
For example, if your system hits a transaction with ‘Smith Enterprises’ as a match to ‘Smith & Co. ’ because it is included in the list of sanctioned entities, could be a case that the second name is AKA/alias of the searched entity.
The misunderstanding arises from the use of the name even when the two are different or common names with distinct meanings.
Outdated AML Data: The Dangers of Faulty Map
Screening with an outdated AML data list is like trying to find your way around a city using a map that has not been updated since the ’90s—chances are, you’ll get lost!
If the AML solution relies on poor AML data, the greater risk lies in missing recently sanctioned information, which could allow potentially high-risk entities to slip through the cracks generating false negatives, or your system could start triggering alerts for customers that are no longer sanctioned thus generating false positives.
The risk doesn’t end here but opens doors for a chain of false positive and false negative risks with potential AML compliance penalties.
Overly Broad Criteria: The Fire Alarm That Never Stops
Imagine having a fire alarm that goes off every time a person barely thinks to prepare dinner. Well, overly broad criteria in AML screening are just as annoying!
When AML solutions set generally overly broad criteria for name screening of entities and individuals, for example, certain profiles exceeding your pre-set compliance criteria are considered suspicious – then the number of alerts increases sharply.
These alerts may include many actual and legal profiles that are not likely to be risky at all.
Lack of Contextual Analysis: The Blind Spot in Name Screening
Consider this as an agent who has an opportunity to go through a news article but only reads the headlines and then concludes the event.
This is what transpires whenever one has no contextual analysis for the name-screening solution!
For instance, your AML solution may fail to capture the organization that could be banned or sanctioned in one jurisdiction but the solution may not show the context hence leading to a quick Sanctions screening false positive.
A lack of contextual analysis can also mean that the solution fails to connect the dots between different pieces of information, potentially missing a genuine threat.
According to a Deloitte AML survey carried out in 2020, financial institutions face a rise in false positive rates by 41% in their current screening results.
Learning the causes of false positives above gives the financial institutions a view on how to improve their AML list screening and cut on the substantial alerts on non-threatening customers and entities thus improving on the evaluation of the genuine risky persons or entities, this appears significant.
Reducing False Positives: A Quick Guide For Financial Institutions
Although sanctions screening false positives can be a major challenge, they can be reduced by comprehending their sources and implementing focused tactics into place.
To improve the accuracy and efficiency of your AML screening procedures, adopt a solution with quality AML Data acting as a strong foundation, strengthened by advanced filters, real-time updates, image matching, contextual analysis, and sophisticated name-matching algorithms.
Here’s entering the AML Watcher in the picture with its all-inclusive AML screening solution that ensures the reduction of maximum false positives and false negatives.
Explore how name-matching screening in combination with image matching allows you to search millions of records in seconds with near-to-zero false positives.
You can get our AML Screening for an exact match at 50% less cost now.
Four Approaches For Near-Zero False Positives: AML Watcher Solution
With its innovative screening features, AML Watcher provides a screening solution to counter all these false positives with tailored risk assessments that enhance the overall risk management process.
Here’s how AML Watcher’s solution helps:
Quality AML Data For Lower False Positives
AML Watcher utilizes quality AML compliance data that is gathered, cleansed, and structured by in-house researchers.
The utilization of advanced filters and biometric screening is everywhere! How does a solution maintain its databases makes all the difference?
AML Watcher’s 60,000+ data sources include originally gathered information from various international and local databases, consolidated sanctioned lists of 200+ domestic and international regimes, structured PEP information in 4 distinct levels, 1300+ disciplinary authority watchlists, and 5000+ global adverse media sources.
Our screening process utilizes the most current information ( readily updated at 15-minute intervals) that keeps track of newly sanctioned or de-listed entities in prominent sanction databases including OFSI, OFAC, MAS, and many more, thus minimizing the false positive rates.
Advanced Name Matching Technology
AML Watcher uses advanced name-matching algorithms that go beyond traditional methods. It uses a combination of match score, phonetic analysis, and filtration based on YOB, unique identifiers, nationality, etc. to enhance the accuracy of name comparisons.
This multi-faceted approach minimizes false positives, ensures that only relevant matches are flagged, reduces the volume of unnecessary alerts, and enhances the efficiency of the screening process.
Biometric Screening
AML Watcher also offers precise biometric screening which combines image matching and name screening to decrease the risks of misidentification and lower the rate of false positives.
One particular characteristic that improves accuracy and reduces detection errors is the individuality of an image.
With less human effort and shorter processing times, this biometric screening ensures accurate results and expedites profile checks.
Customizable Screening Parameters
AML Watcher offers tailored alert thresholds, that enable institutions to customize their screening settings to meet their specific requirements and risk profile.
- Adjust the sensitivity of the screening system to balance between identifying actual risks and minimizing false positives.
- Financial institutions can set specific parameters based on industry requirements, regional regulations, or internal policies. Define your custom risk scoring criteria to lower maximum false positives.
So, in the end, we come to the point that one of the essential issues in the constantly developing field of AML compliance is the problem of raising false positives and finding reliable ways of the AML false positive reduction.
By utilizing these advanced screening features backed by machine learning and artificial intelligence, AML Watcher helps financial institutions focus on real threats, streamline their compliance procedures, and lower false positives.
Our strategy is to discover a balance between this sensitivity and risk by reducing false positives and making sure that valid names or authentic profiles are not mistakenly recognized as sanctioned, thereby filtering danger without jeopardizing credible clients.
Our updated global watchlist, sanction lists, and adverse media list allow you to search millions of records in seconds with near-to-zero false positives at 50% less cost.
Contact us now to get a free demo or a call back from our experts to learn more about the features of the AML screening solution.
Book Free DemoRelated Articles
We are here to consult you
Switch to AML Watcher today and reduce your current AML cost by 50% - no questions asked.
- Find right product and pricing for your business
- Get your current solution provider audit & minimise your changeover risk
- Gain expert insights with quick response time to your queries